Face Detection through Scale-Friendly Deep Convolutional Networks
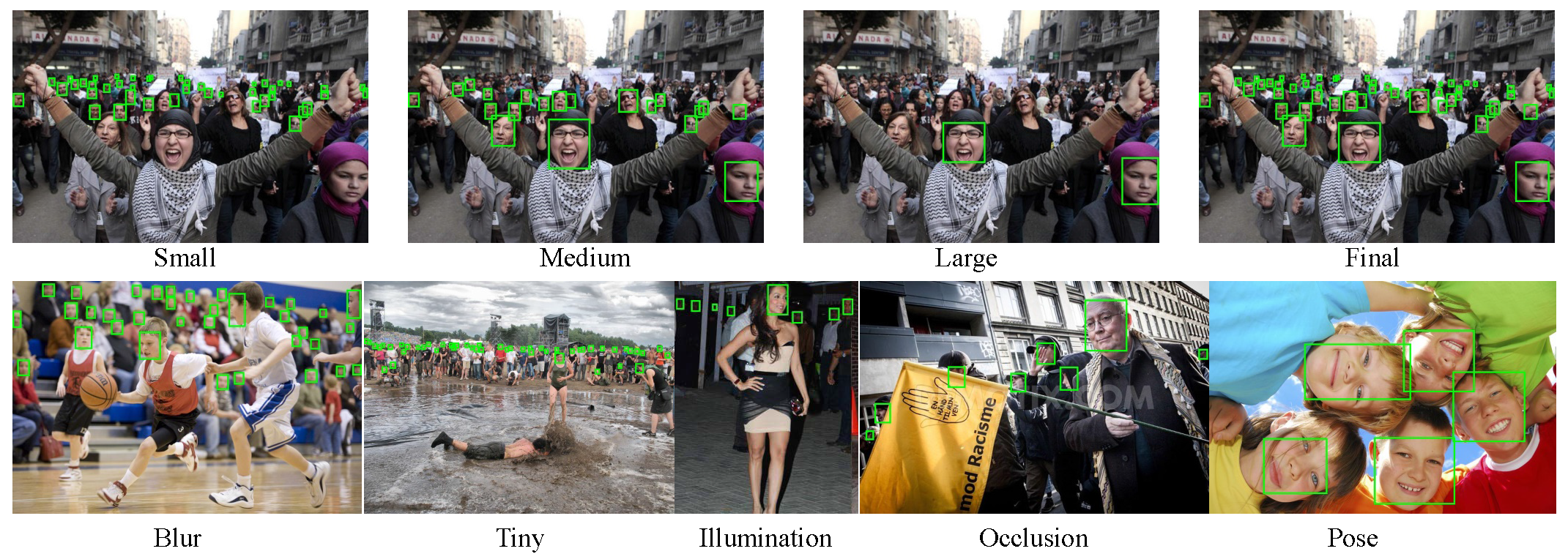
Abstract
In this paper, we share our experience in designing a convolutional network-based face detector that could handle faces of an extremely wide range of scales. We show that faces with different scales can be modeled through a specialized set of deep convolutional networks with different structures. These detectors can be seamlessly integrated into a single unified network that can be trained end-toend. In contrast to existing deep models that are designed for wide scale range, our network does not require an image pyramid input and the model is of modest complexity. Our network, dubbed ScaleFace, achieves promising performance on WIDER FACE and FDDB datasets with practical runtime speed. Specifically, our method achieves 76.4 average precision on the challenging WIDER FACE dataset and 96% recall rate on the FDDB dataset with 7 frames per second (fps) for 900 * 1300 input image.
Downloads
Citation
@article{yang2017scaleface, title={Face Detection through Scale-Friendly Deep Convolutional Networks}, author={Yang, Shuo and Xiong, Yuanjun and Loy, Chen Change and Tang, Xiaoou}, journal={arXiv preprint arXiv:1706.02863}, year={2017} }