From Facial Parts Responses to Face Detection: A Deep Learning Approach
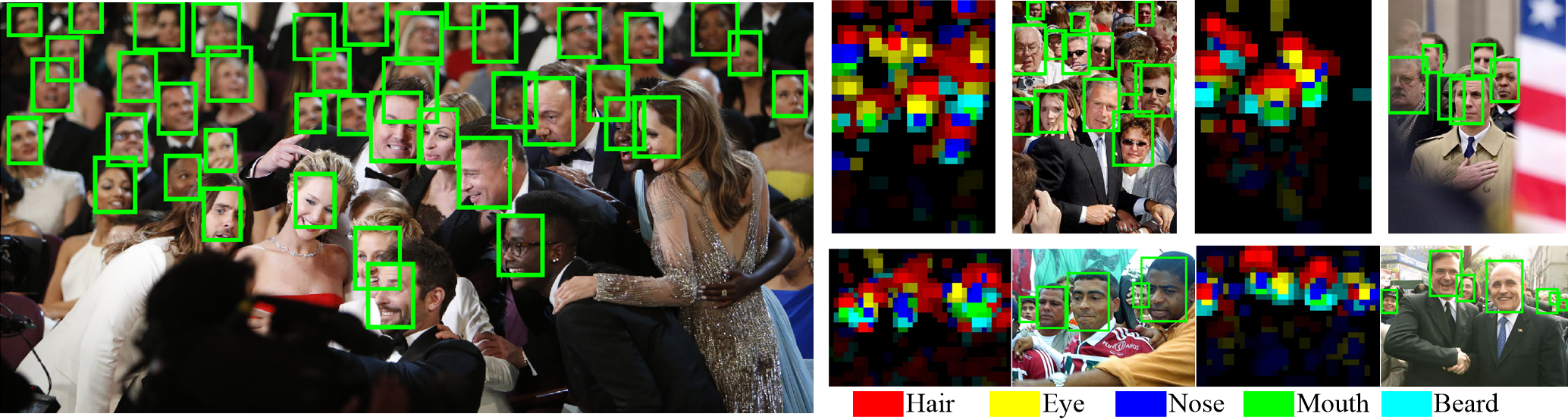
Abstract
In this paper, we propose a novel deep convolutional network (DCN) that achieves outstanding performance on FDDB, PASCAL Face, and AFW. Specifically, our method achieves a high recall rate of 90.99% on the challenging FDDB benchmark, outperforming the state-of-the-art method by a large margin of 2.91%. Importantly, we consider finding faces from a new perspective through scoring facial parts responses by their spatial structure and arrangement. The scoring mechanism is carefully formulated considering challenging cases where faces are only partially visible. This consideration allows our network to detect faces under severe occlusion and unconstrained pose variation, which are the main difficulty and bottleneck of most existing face detection approaches. We show that despite the use of DCN, our network can achieve practical runtime speed.
Demo
Downloads
Citation
@inproceedings{yang2015faceness, author = {Shuo Yang, Ping Luo, Chen Change Loy, and Xiaoou Tang}, title = {From Facial Parts Responses to Face Detection: A Deep Learning Approach}, booktitle = {Proceedings of International Conference on Computer Vision (ICCV)}, month = December, year = {2015} }